Introducing the Continuous Impact Loop
The Continuous Impact Loop by Headway. A new way to approach continuous discovery and delivery for product teams at high-growth SaaS startups.
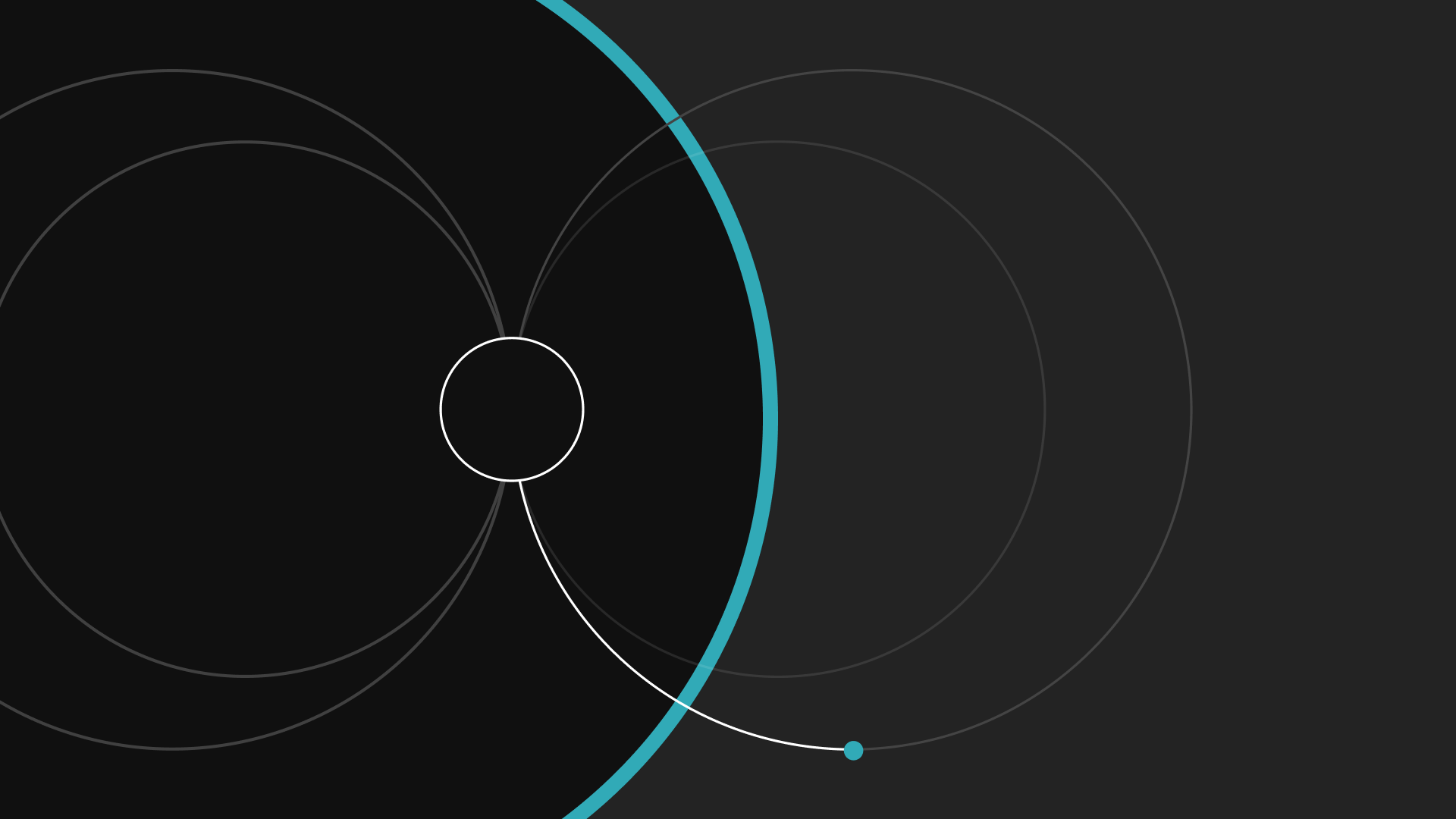
The why
Does your product team feel like a feature factory?
You’re launching new features, but after you ship - there’s still not enough impact on growth.
So what are your options?
Before we introduce our Continuous Impact Loop, let’s talk about its foundations.
Continuous discovery and delivery methods
You may be hearing from amazing product leaders like Teresa Torres, Marty Cagan, Melissa Perri (among many others) on how to make better product teams and focusing on your customers to find growth opportunities.
Believe the hype. We are fans and absolutely believe in the huge impact it can make for any startup.
What’s missing
Problem is there are so many aspects of what you should do, how you should work… it can be overwhelming. OKR’s, Outcomes vs. Outputs, Continuous Discovery, Continuous Delivery, Dual Track Agile, you’ve heard it all.
How do they all fit together? Is there a model that brings them all together? What would it look like to work in such a team?
What are the stages in the learning lifecycle? How might product, design, engineering collaborate at each stage?
How do we measure our team’s impact?
How might continuous discovery habits merge with continuous delivery methods into a repeatable growth process?
In essence
How should the best product teams operate?
The Continuous Impact Loop
We are introducing a growth model for how some of the best product teams work to drive business results.
We call it the Continuous Impact Loop, a unified approach to continuous discovery and delivery.

So many teams don’t operate this way - which is why we feel it needs to be written more about.
Instead of focusing on simply delivering the next feature on the product roadmap, next-level product teams come together around a clear product metric and run continuous feedback loops through both discovery and delivery to make forward progress.
You no longer focus on shipping to production. That's table stakes.
Stop just shipping. Start making impact.
We call it the Continuous Impact Loop. Get ready to make waves.
Your product team’s new guiding light
Continuous Impact is a series of fast feedback loops, all orbiting around your central product metric.
Continuous Impact has four repeatable loops:
- Explore
- Prototype
- Pilot
- Scale Up
You begin with the exploration phase and learn more about what opportunities and problems your product team needs to solve. Then you’ll begin prototyping with customers to get feedback. From there you begin to pilot-test product changes in-market before expanding the loop and finally, scaling up.
Before you can apply Continuous Impact
Your team's mindset and structure matters.
Prerequisites for Continuous Impact:
- You realize that being a Feature Factory won’t solve for your growth
- You have moved to Empowered Teams
- You realize how Empowered Teams changes everything about how your teams operate (autonomous, cross-functional, impact-chasing) and the leadership behavior changes required to enable this
- You know how to set good product metrics, also called OKR’s – but actually implemented correctly with Empowered Teams. This is difficult to do well
Video Presentation
We have everything about the Continuous Impact Loop laid out below for you here in this blog post, but you can also watch our presentation on our model here:
First step: Setting the product metric
Product metrics define success for the team and address a critical business bottleneck.
Solid product metrics measure things like customer behavior, time to value, latency, and more. Whatever metric you select - focus on leading indicators to business impact, rather than business-level metrics like revenue or internal metrics like agile velocity.
The problem
Let’s say you run a data visualization product and you’re charged with improving new customer retention. Measuring retention isn’t good enough - because it’s a lagging indicator. You need to find a leading indicator.
Finding a leading indicator
Maybe you’re finding low activation and engagement, where many new customers churn early and fail to complete the onboarding process. Most of them don’t get to the “magic moment” where they experience the best value of your product and fully realize how your product can help them.
Experiencing this “magic moment” faster can help customers see the value and drive engagement / retention. In this example, your team decides the “magic moment” is when a new user is able to view their first visualization with their own business data.
Establishing a baseline
The first thing to do is to measure a baseline for this magic moment.
- How many new customers per month get beyond this point currently? (Conversion Rate)
- How long does it take them? (Time to Value)
Setting the metric
This magic moment becomes your team’s metric. You want to get more new customers to experience this moment as fast as possible. You could opt to measure it in time-to-value if speed is the biggest hurdle, or in conversion rate percentage, or combine them.
Example
Increase new customers seeing their first data visualization within 24 hours from 20% to 80%
Whatever target condition you select for your team, make sure it’s exciting enough to help your team get inspired and thinking differently, but not too far out of reach to make it impossible to achieve.
Explore - Loop 01
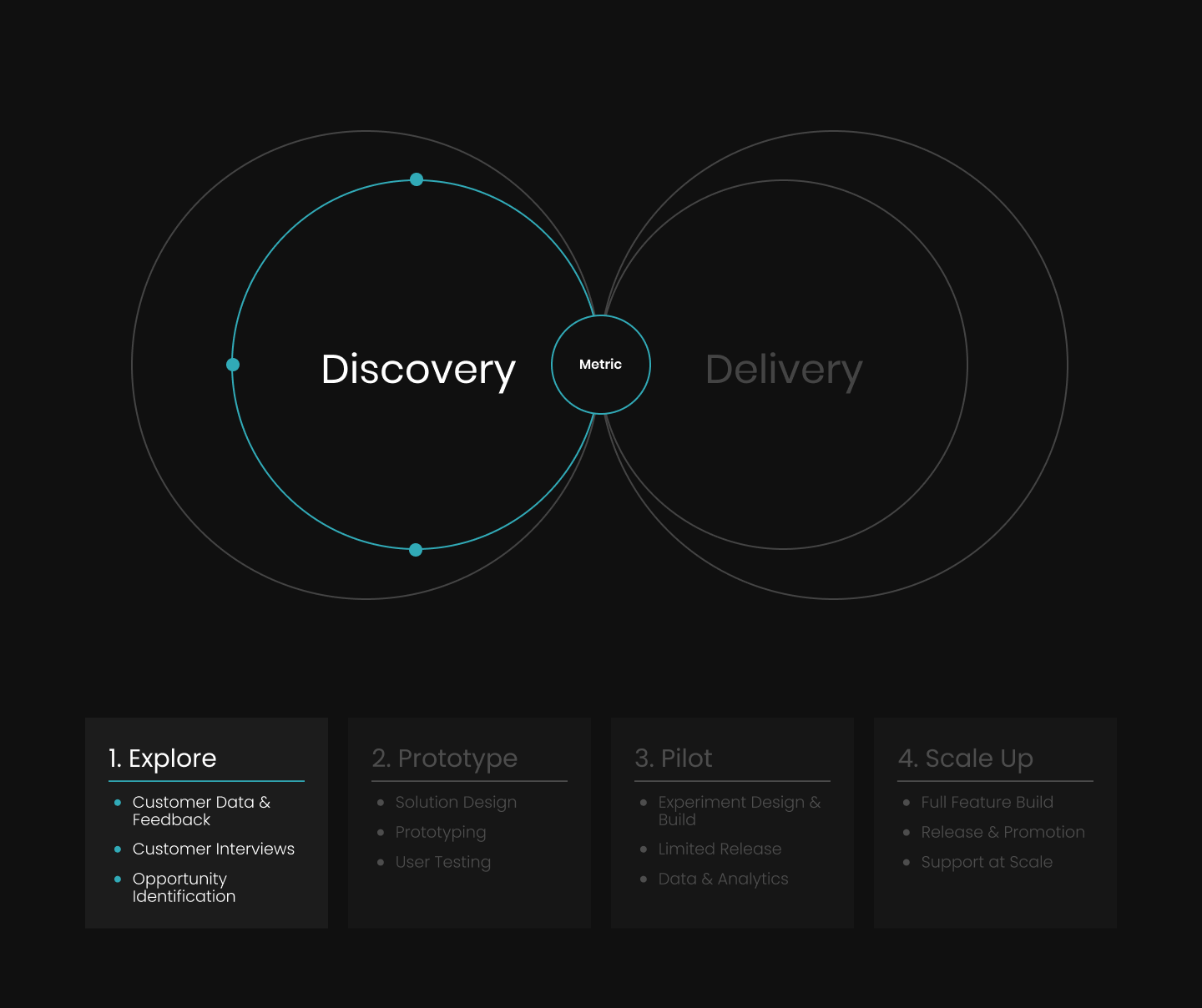
Critical Question
How might we find opportunities that will have the most impact on our product metric?
Now that you have the metric in place, you need to find out how to move that needle.
Where should your team focus their energy?
In the Explore loop, you’ll be discovering and deciding on the best opportunities that your team can chase.
Customer data and feedback
You probably already have a lot of signals coming at you.
A great place to often start is by looking at the analytics data you already have in front of you.
Examples of good questions to ask yourself here are:
- Who is getting stuck?
- Who is making it past the magic moment?
- Where do they get stuck?
- What are they seeing when they disengage?
You probably also are getting signals from sales, feedback forms, and from customer success / support.
Examples of good questions to ask yourself here are
- What are the themes and patterns of the feedback?
- What aren’t we hearing about?
- Where is this feedback coming from and how might it be biased / unreliable?
Many teams stop right here and move into shipping features. We believe that’s wrong. These data and feedback signals may provide you with themes, but it will not provide you with fast validation. You may find who’s affected most and where they fall off before this magic moment, but...
Data only tells half the story
Data is great, but data only tells you WHAT is happening - It can’t explain WHY. You may find themes, but you don’t know if that’s really the best opportunity to chase at this time. You need to find what is causal, not just correlated.
Once you have a theme about who and where the opportunities might lie, it’s time to move onto customer discovery to dive deeper.
Customer interviews
We talk so often about numbers and SaaS metrics… sometimes we forget that we are building products for real people. People are complex, and the answer is almost never as simple as we think. The real answers you seek are found in customer’s stories. Stories are gold, and they provide the missing context you’ll never get from your data, sales team, or support team.
It starts by asking a critical research question.
Example:
“What’s the difference between customers who get stuck vs those that make it through?”
Your research question will lead you to recruiting. Who should we recruit to learn about this? Getting customers on a call with you is key so you can learn quickly.
Teresa Torres talks about this a lot more in-depth with Continuous Discovery. We suggest automated customer interview recruitment so you have a regular cadence of customers to speak with and can answer your discovery questions faster.
Examples of good questions you might ask in the interviews
- When was the last time you had X problem?
- What were you trying to achieve?
- What did you do instead?
- What worked well for you? What didn't work well?
- What did you do immediately before and after?
- Who else was involved and what is their role?
Realize that these questions are a simple starting point and change completely depending on the research question you’re trying to answer. Get some great conversations going and dive deep into their stories.
Opportunity identification
You now have deep customer interview insights + quant data to start mapping the opportunity space. This is a synthesis exercise. Start by viewing your notes and sketches from your customer interviews.
Example questions to observe for
- What was causal to their behavior?
- When did they get emotional?
- What was surprising?
- What didn’t they say?
You’ll want to build an Opportunity Tree here, which provides a relationship of opportunities that map back to your product metric.
Look for patterns and themes
A simple way to start building your tree is by moving backwards from Solutions (which come up naturally) to Opportunities by asking: What opportunity/problem does this solution solve?
Invite your team to re-frame the problems in new ways and re-draw the tree.
Examples of good questions to answer
- What patterns did we encounter across our customer interviews?
- What common problems did we see?
- What solution ideas come to mind?
- Which opportunity do each of those solutions solve for?
- How do these (or don’t these) map back to our product metric?
- What core assumptions are we making about our target customer(s)?
- Which opportunities are likely dead-ends?
- Which opportunities are likely to have the biggest impact?
You very well may find that opportunities you thought were real - are really not a path to moving the current needle.
Once you have a map of the opportunities, you’ll need to prioritize them and decide where to zoom-in. You can’t solve all the opportunities at once. Select an opportunity area that will have the biggest potential impact & emphasize speed. Now you’re ready to begin prototyping.
Prototype - Loop 02
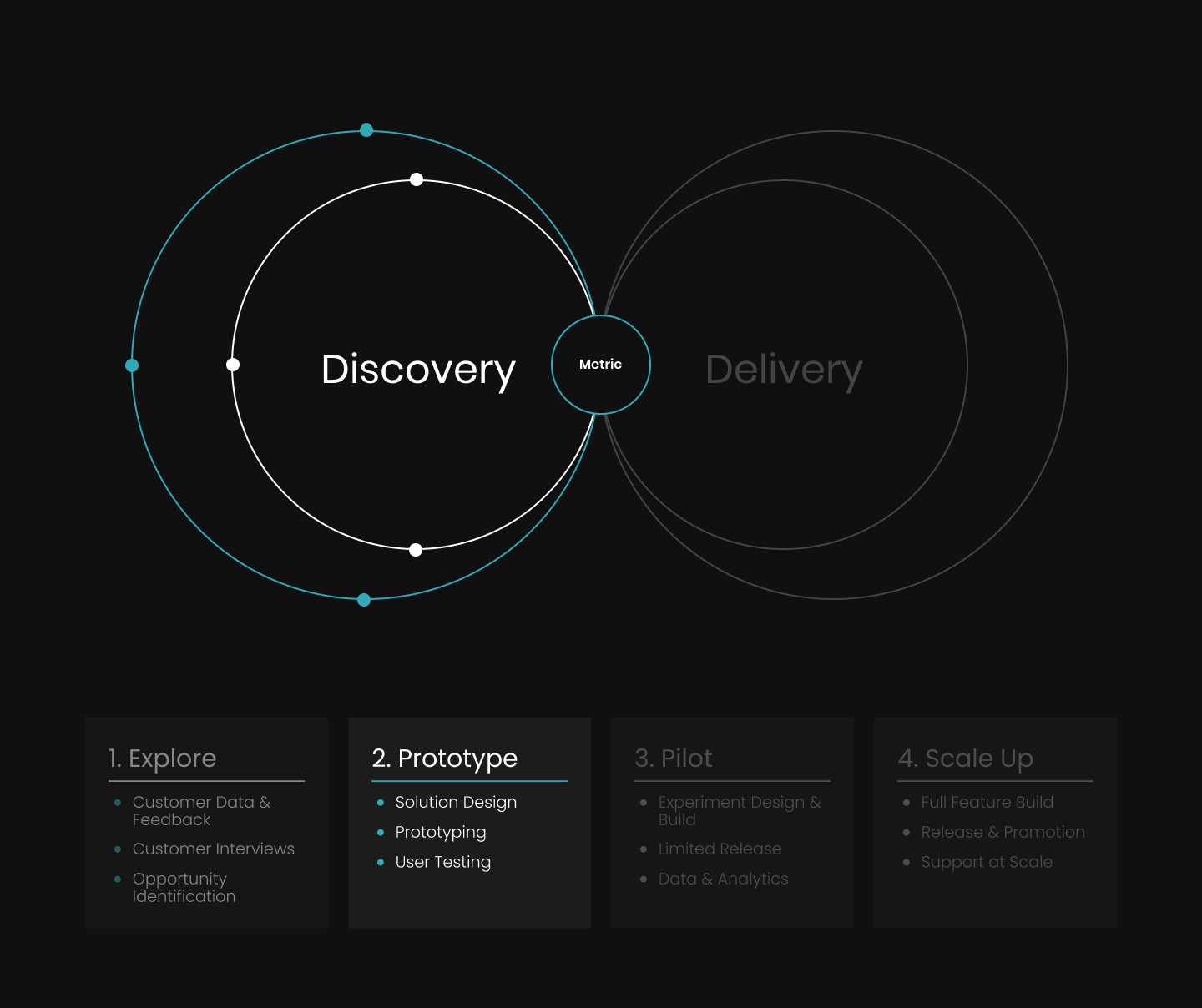
Critical question
How might we design and test solutions that have a high likelihood of solving the top opportunities?
Prototyping isn’t something you just do for new products, it’s something you should also be doing to improve existing experiences and exploring how well certain solutions might solve for your product metric.
Solution design
Start by ideating multiple solutions for the top opportunity you selected. This starts with a divergent exercise, and you can even get your whole team involved. Examples of good questions to ask here are:
- How might we solve this - in the simplest way possible?
- How might we solve this - while creating customer delight?
- How might we solve this - in the best customer experience?
- How might we solve this - using the latest technology?
- How might we solve this - if we had unlimited funds?
- How might we solve this - if we were kindergarteners?
- How might we solve this - if we were rocket scientists?
- How might we solve this - if we were: Google? Apple? Amazon? Netflix?
Remember
This is about speed to learning. So while this is fun, don’t get caught up in going too deep. Feel free to introduce time constraints. Plays to reach for here are customer experience mapping and low-fidelity sketches.
Select a couple of the best winners to move forward with.
Before you move onto prototyping, peel back the solution concepts you’ve outlined and answer this key question:
What assumptions are we making in this solution?
What you’ll really want to move forward with is designing prototypes to test those underlying assumptions.
Prototyping
The key here is to move multiple solutions into prototyping. Why multiple solutions? Because learning happens in the contrast. We don’t just want to learn if one solution works. That’s a yes/no answer, and you can’t learn much in binary world. We want to learn which of multiple solutions solves the problem better and why. We can’t learn what we don’t test.
Examples of prototypes might include:
- Clickable prototypes
- Landing pages
- Ad designs
- CTA changes
- Simulated Shopping
- Front-end app with mocked data, no backend
- Etc.
We often reach for Figma here to get multiple prototypes ready. If you’re using a Design System, these prototypes can come together very fast. Design systems are a great investment not only for your engineering team, but also to help speed up discovery through rapid reusability.
There is an argument to be made for either low-fidelity or high-fidelity prototypes.
The question is
What’s the fastest way to get the learning you need?
Low fidelity prototypes
We’ve found lower-fidelity to be most useful when contrasting a general direction, and allows the customers to co-create with us from their own interpretation and leverage the customer’s imagination.
Pros
Leverages imagination
Cons
Skips [potentially important] details
High fidelity prototypes
We’ve found higher-fidelity to be most useful when you need to to learn about imagined use and details. For instance: missing fields they need to see, how advanced search filtering works, etc. High fidelity prototypes also leverages the customer’s emotions as it feels very real. We’ve even had people cry in prototype interviews with high fidelity prototypes.
Pros
Detailed feedback, leverages emotion, useful for sales & pre-sales
Cons
Less co-creation, time to create without a design system
User testing
Now that your prototypes are ready, it’s time to get outside the building and test them with real customers.
This all comes back to this key question: What are you trying to learn?
It’s important to design your user tests around some key learning.
For example
- Usability - Time to complete a flow
- Perception Test - testing for understandability
- Delight - Emotional response
- Desirability - test for some form of currency (Relational, Data, Monetary)
- Etc.
Once again you’ll recruit your target customers, and conduct your testing. How many customers do we need to test with? That depends on your product and what you’re trying to learn. If your learning is focused enough, surprisingly you often only need a handful of customers to provide you feedback to know if you’re headed in the right or wrong direction.
In your testing you’ll want to have the customer contrast one solution vs. another.
- Which solution did they like best? Why?
- What elements did they find most helpful / valuable? Why?
- What was confusing?
- What is missing? What is not needed?
- What would they change? How would they re-imagine it?
Hopefully you find this rapid feedback as incredible as we do. Speed to learning beats building the wrong thing every time.
Pilot - Loop 03
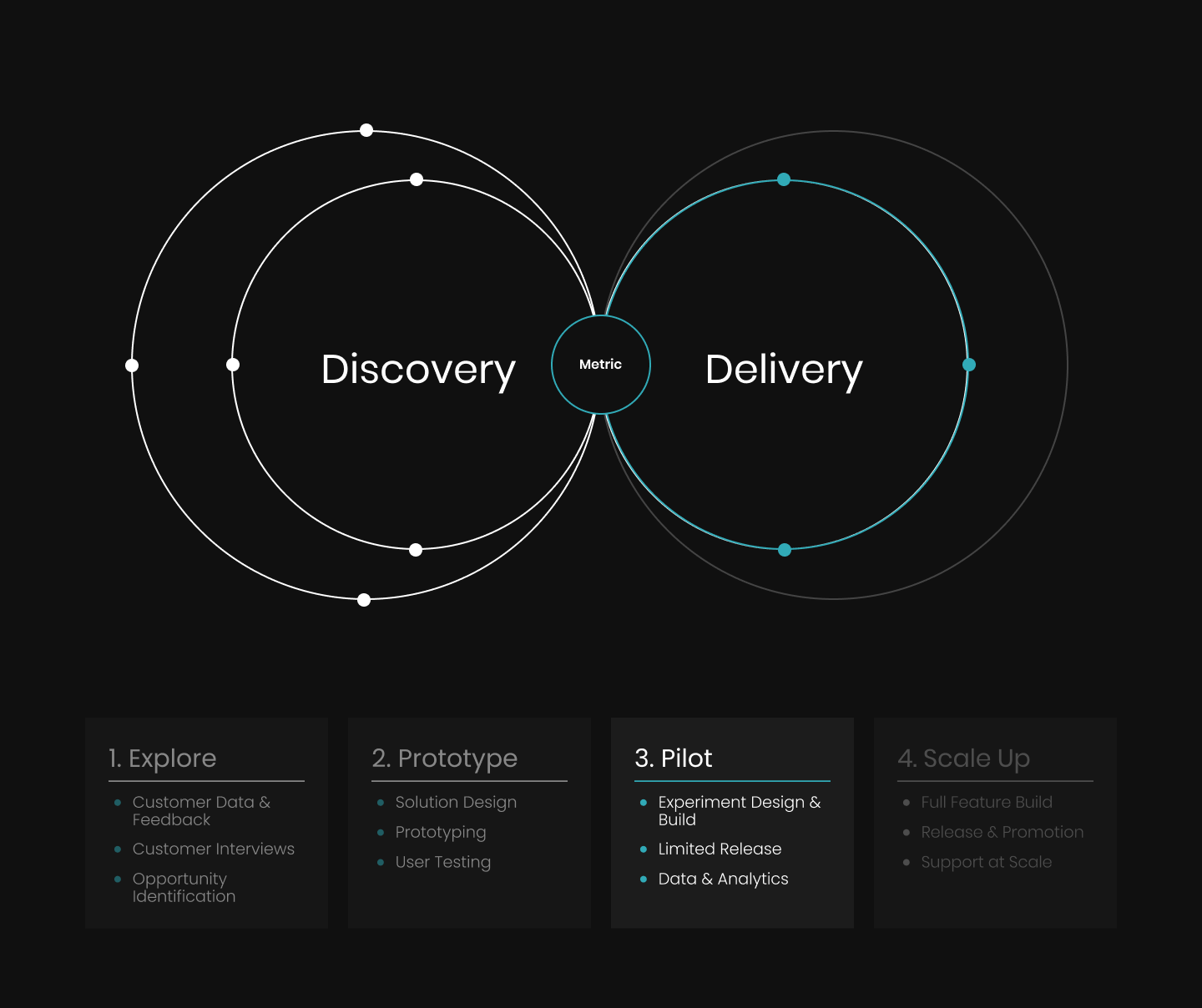
Critical question
How much will our solution(s) actually move our metric in the real world?
Once your prototype has been tested and validated, it’s now time to roll-out a real in-product pilot with customers in the real world.
Experiment design and build
The first thing to do is design your experiment. It’s important to setup your experiment criteria before launching the pilot. You don’t want to run the pilot with all your customers. This is a test, so you'll need a control group and a test group. You want to balance speed to learning with limiting experiment size to protect both customers and yourself if things go awry.
Questions for you to consider
- How will we know when the feature has failed to move the needle?
- What data do we need to track / instrument in order to know if it’s working?
- Who would benefit most from this pilot?
- Who should not see this new feature or capability?
- What risks does this pose?
- How do we mitigate those risks?
- Will this be an A/B test between the pilot and everyone else? A/B test within the pilot?
- How long should this test run? When will we have enough data to make a decision?
Designing a good experiment will help you decide later if the feature is worth keeping or should be tossed. The worst thing you can do is keep all these experiments in the product - increasing the burden on the customer and increasing your team’s maintenance burden.
You want to be mindful of an indicator that lets you know whether it is working or failing to have the impact on your product metric.
Limited release
Now that your team has designed the experiment and built the feature, it’s time to do a limited release to your pilot customers.
A really important engineering capability here is called Feature Flags. This is the ability to turn certain features on and off for certain customers in the product. In other words, you have different product capabilities enabled for different customers. This goes far beyond pricing tiers, but extends all the way into a database of experiments being run and which customers are in each experiment.
You’ll want to make sure quality is high and you haven’t produced any negative side effects when the pilot launches.
Data and analytics
Now that you have a subset of real customers in a pilot with your new feature / capability, it’s time to look at the data. You should have all the data streaming into your analytics. It’s very important to be able to segment your analytics so that you can see your pilot group separately from your other customers. Examples of good questions to ask here to help you evaluate success or failure:
- How many customers in the pilot were exposed to the feature / capability?
- How did the pilot group’s behavior differ from our other customers?
- Were we able to move our product metric?
- What was the experience like for our pilot customers?
- What did they like? What didn’t they like?
- Who made it through and how far beyond that did they get?
- Who got stuck and why might that be?
Based on what you learned, it’s very plausible you would go back to the Explore loop to learn more about what worked, what didn’t, and why.
You may decide here to kill the feature if it fails to meet exceed your failure criteria.
Reasons to kill failed features and remove them from the codebase
- Burden your dev team with maintenance
- Burden your design team with thicker information architecture
- Burden customers with things they won’t use
- Burden your support team with documentation baggage
Failure is ok. Removing failed experiments from the product is actually a good thing. The key is to iterate faster and get to learning - so you can find what actually works.
If the experiment was successful, then it’s time to scale it up.
Scale - Loop 04
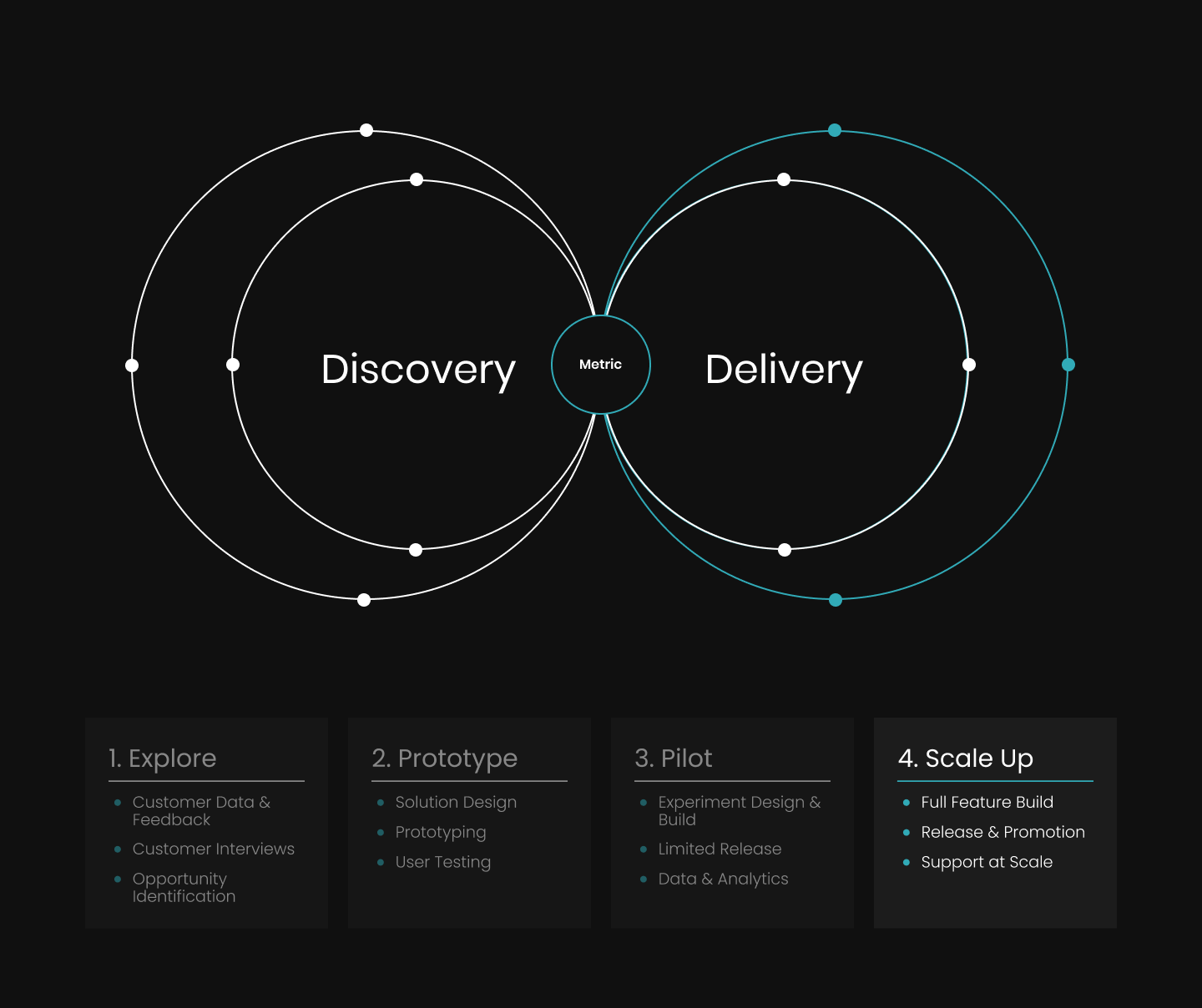
Critical question
How might we scale up what’s proven to work?
Now that you have an experiment that’s proven to move the needle, and help customers get further in their journey, it’s time to scale it up to the rest of your customer base.
Full feature build
After the pilot run, any feedback from users can then be implemented. This is where we add missing pieces and functionality to be prepared for full release. You probably realized there were missing capabilities that you need to add, or additional scenarios to take into consideration. Your design team probably would like to smooth out some bumps in the experience. Your dev team probably has some automated testing to add, or architecture changes to make to ensure it can scale properly with minimum technical debt. There’s usually work to be done before flipping the switch and releasing to all your customers.
Release and Promotion
While the feature is being built, you are also now preparing for release. This is when you are communicating delivery expectations to your marketing, sales, and customer success teams. Perhaps creating demos for upcoming presentations or a user conference. You’ll be getting education and technical documentation changes formalized. And you’ll be thinking about how this affects your marketing messaging and any potential promotional campaigns. Maybe it’s a small tweak and doesn’t need to be promoted. Or you prefer a staggered release plan, so the feature will roll out over several days to ensure success. After the full feature is built, tested, and ready - it’s time to release and roll it out.
Support at Scale
It’s important to be able to support your product and feature releases at scale. Make sure you have the people in place to be able to support across your customer base. Questions you’ll want to think about here include:
- Is our customer success team being overwhelmed with aspects of the release?
- Which customers still need more support? And why?
- How do we make self-serve work better for customers?
- How will we know if our education / documentation is working?
- What’s our response time to customers?
- How does escalation work?
The goal is to have mechanisms in place to answer questions customers have, reduce confusion, capture feedback your team needs, and provide assistance at scale (without overwhelming your support team).
Progress is not linear
Realize that this is an iterative & interactive process - not a linear process. Any loop can start a different loop. For instance, after Pilot - you may go back to Explore, or go back to Prototyping alternative solutions, or pivot and run a different in-product Pilot experiment – all before you Scale Up.
Metric focus until the goal is met
These efforts across product, design, and development leads right back to the metric. Each loop creates a clear guide for your team to follow. With this clear alignment, you create empowered teams that are designed to impact the growth of the business, not just ship outputs.
Stay focused on the leading indicator and loop until you’ve made impact.
Find the next metric to impact
As we’ve shared in our Ready to Scale video series, once you fix one metric or growth bottleneck, the problems shift into another part of the customer journey. Maybe you’ve unlocked paid user activation, but now retention is starting to get worse.
Define the next best metric to impact, and go back to the Explore loop. Just like last time, create alignment with your team around that metric and repeat the process.
Feedback welcomed
We hope you enjoyed learning about the Continuous Impact Loop. Start applying it and please let us know how it’s working for you. You can contact us here with any feedback or questions.